Accurate differentiation of pathological subtypes of renal tumour by applying a machine learning model to epigenetic markers
BAUS ePoster online library. Rossi S. 06/23/21; 319030; p12-2
Disclosure(s): nil
Ms. Sabrina H. Rossi
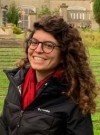
REGULAR CONTENT
Login now to access Regular content available to all registered users.
Abstract
Discussion Forum (0)
Rate & Comment (0)
Introduction:
Small renal masses (SRMs) represent a diagnostic challenge, with 20% of SRMs found to be benign post-operatively. We developed and validated a machine learning (ML) model, leveraging DNA methylation analysis in >1500 patient samples, to classify pathological subtypes of renal tumours and improve diagnosis.
Methods:
Methylation was evaluated using the Illumina 450k microarray and EPIC Methyl Capture sequencing platforms. We combined our own dataset and TCGA data (N=approx 158000 markers). This dataset was divided into training and testing sets, using 4-fold cross validation, and used to train an XGBoost model to classify samples into one of five pathological subtypes. External validation was performed on two independent datasets (Brennan et al and Chopra et al).
Results: The integrated dataset consisted of 1234 samples, including: 463 ccRCC, 303 pRCC, 93 chRCC, 61 oncocytomas and 314 normal kidney. The prediction accuracy in the testing set was 0.954; with high class-wise ROC AUCs: 0.995 (ccRCC), 0.995 (pRCC), 0.986 (chRCC), 0.999 (oncocytoma), 0.999 (adjacent normal). This model was externally validated on 245 ex-vivo tissue biopsies from 100 renal tumours. The accuracy was 0.829 and average class-wise ROC AUCs were: 0.978 (ccRCC), 0.945 (pRCC), 0.993 (chRCC), 0.917 (oncocytoma), 0.971 (adjacent normal). The impact of methylation heterogeneity on our classifier was assessed by evaluating 97 multi-region samples from 18 ccRCC patients.
Conclusion:
A ML model based on DNA methylation can differentiate renal tumours with excellent accuracy, including external validation. The model was applied to renal biopsies and has the potential to improve the diagnostic pathway.
Small renal masses (SRMs) represent a diagnostic challenge, with 20% of SRMs found to be benign post-operatively. We developed and validated a machine learning (ML) model, leveraging DNA methylation analysis in >1500 patient samples, to classify pathological subtypes of renal tumours and improve diagnosis.
Methods:
Methylation was evaluated using the Illumina 450k microarray and EPIC Methyl Capture sequencing platforms. We combined our own dataset and TCGA data (N=approx 158000 markers). This dataset was divided into training and testing sets, using 4-fold cross validation, and used to train an XGBoost model to classify samples into one of five pathological subtypes. External validation was performed on two independent datasets (Brennan et al and Chopra et al).
Results: The integrated dataset consisted of 1234 samples, including: 463 ccRCC, 303 pRCC, 93 chRCC, 61 oncocytomas and 314 normal kidney. The prediction accuracy in the testing set was 0.954; with high class-wise ROC AUCs: 0.995 (ccRCC), 0.995 (pRCC), 0.986 (chRCC), 0.999 (oncocytoma), 0.999 (adjacent normal). This model was externally validated on 245 ex-vivo tissue biopsies from 100 renal tumours. The accuracy was 0.829 and average class-wise ROC AUCs were: 0.978 (ccRCC), 0.945 (pRCC), 0.993 (chRCC), 0.917 (oncocytoma), 0.971 (adjacent normal). The impact of methylation heterogeneity on our classifier was assessed by evaluating 97 multi-region samples from 18 ccRCC patients.
Conclusion:
A ML model based on DNA methylation can differentiate renal tumours with excellent accuracy, including external validation. The model was applied to renal biopsies and has the potential to improve the diagnostic pathway.
Introduction:
Small renal masses (SRMs) represent a diagnostic challenge, with 20% of SRMs found to be benign post-operatively. We developed and validated a machine learning (ML) model, leveraging DNA methylation analysis in >1500 patient samples, to classify pathological subtypes of renal tumours and improve diagnosis.
Methods:
Methylation was evaluated using the Illumina 450k microarray and EPIC Methyl Capture sequencing platforms. We combined our own dataset and TCGA data (N=approx 158000 markers). This dataset was divided into training and testing sets, using 4-fold cross validation, and used to train an XGBoost model to classify samples into one of five pathological subtypes. External validation was performed on two independent datasets (Brennan et al and Chopra et al).
Results: The integrated dataset consisted of 1234 samples, including: 463 ccRCC, 303 pRCC, 93 chRCC, 61 oncocytomas and 314 normal kidney. The prediction accuracy in the testing set was 0.954; with high class-wise ROC AUCs: 0.995 (ccRCC), 0.995 (pRCC), 0.986 (chRCC), 0.999 (oncocytoma), 0.999 (adjacent normal). This model was externally validated on 245 ex-vivo tissue biopsies from 100 renal tumours. The accuracy was 0.829 and average class-wise ROC AUCs were: 0.978 (ccRCC), 0.945 (pRCC), 0.993 (chRCC), 0.917 (oncocytoma), 0.971 (adjacent normal). The impact of methylation heterogeneity on our classifier was assessed by evaluating 97 multi-region samples from 18 ccRCC patients.
Conclusion:
A ML model based on DNA methylation can differentiate renal tumours with excellent accuracy, including external validation. The model was applied to renal biopsies and has the potential to improve the diagnostic pathway.
Small renal masses (SRMs) represent a diagnostic challenge, with 20% of SRMs found to be benign post-operatively. We developed and validated a machine learning (ML) model, leveraging DNA methylation analysis in >1500 patient samples, to classify pathological subtypes of renal tumours and improve diagnosis.
Methods:
Methylation was evaluated using the Illumina 450k microarray and EPIC Methyl Capture sequencing platforms. We combined our own dataset and TCGA data (N=approx 158000 markers). This dataset was divided into training and testing sets, using 4-fold cross validation, and used to train an XGBoost model to classify samples into one of five pathological subtypes. External validation was performed on two independent datasets (Brennan et al and Chopra et al).
Results: The integrated dataset consisted of 1234 samples, including: 463 ccRCC, 303 pRCC, 93 chRCC, 61 oncocytomas and 314 normal kidney. The prediction accuracy in the testing set was 0.954; with high class-wise ROC AUCs: 0.995 (ccRCC), 0.995 (pRCC), 0.986 (chRCC), 0.999 (oncocytoma), 0.999 (adjacent normal). This model was externally validated on 245 ex-vivo tissue biopsies from 100 renal tumours. The accuracy was 0.829 and average class-wise ROC AUCs were: 0.978 (ccRCC), 0.945 (pRCC), 0.993 (chRCC), 0.917 (oncocytoma), 0.971 (adjacent normal). The impact of methylation heterogeneity on our classifier was assessed by evaluating 97 multi-region samples from 18 ccRCC patients.
Conclusion:
A ML model based on DNA methylation can differentiate renal tumours with excellent accuracy, including external validation. The model was applied to renal biopsies and has the potential to improve the diagnostic pathway.
Code of conduct/disclaimer available in General Terms & Conditions
{{ help_message }}
{{filter}}