A machine learning study of post-ureteroscopy infectious complications
BAUS ePoster online library. Geraghty R. 06/23/21; 319057; p14-9
Disclosure(s): I am an academic clinical fellow supported by NIHR
Mr. Robert Geraghty
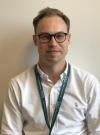
REGULAR CONTENT
Login now to access Regular content available to all registered users.
Abstract
Discussion Forum (0)
Rate & Comment (0)
Introduction:
Post ureteroscopic infectious complications have proven difficult to predict. We therefore aimed to use described risk factors to delineate low and high risk groups to compare risk of post ureteroscopic infective complications.
Materials and Methods:
Retrospective cohort of all patients undergoing ureteroscopy from June 2019 to September 2019. Data collected on age, sex, type of admission, presence of a stent, operative factors and the presence of risk factors (diabetes mellitus, neurological disorders, immunocompromised state, abnormal upper tract anatomy, previous reconstructive surgery, previous post-operative UTI). Patients were divided into low(no risk factors)/high risk groups. Adverse outcome defined as post-operative pyrexia, ITU/HDU admission for urosepsis and/or 30 day readmission for infective symptoms. Binary logistic regression (BLR) and random forests (RF) were used for model construction.
Results:
There were 258 patients (female, n=96, male, n=162; low-risk, n=28, high-risk, n=230), with a mean age of 60±17 years. Adverse outcomes: low-risk, n=4(14.3%)l high-risk, n=34 (14.7%).
The non-validated BLR model demonstrated three significant predictive factors: neurological disorder (p=0.03), stent on strings insertion (p=0.04) and complete stone clearance (p=0.04). Model accuracy=0.90 (95% CI:0.85-0.93), sensitivity=0.90, specificity=0.80. There were no significant differences between the low/high risk groups (p=0.14).
The internally validated RF model demonstrated two significant predictive factors (history of post-operative UTI, p=0.004; neurological disorder, p<0.001). Model accuracy=0.88 (95% CI: 0.77-0.94), sensitivity=1.00, specificity=0.00, AUC=0.71. Figure for literature comparison.
Conclusions:
Presence of a neurological disorder is consistent risk factor for post-ureteroscopy infective complications. Modern machine learning techniques fail to accurately predict those with an infective complication.
Post ureteroscopic infectious complications have proven difficult to predict. We therefore aimed to use described risk factors to delineate low and high risk groups to compare risk of post ureteroscopic infective complications.
Materials and Methods:
Retrospective cohort of all patients undergoing ureteroscopy from June 2019 to September 2019. Data collected on age, sex, type of admission, presence of a stent, operative factors and the presence of risk factors (diabetes mellitus, neurological disorders, immunocompromised state, abnormal upper tract anatomy, previous reconstructive surgery, previous post-operative UTI). Patients were divided into low(no risk factors)/high risk groups. Adverse outcome defined as post-operative pyrexia, ITU/HDU admission for urosepsis and/or 30 day readmission for infective symptoms. Binary logistic regression (BLR) and random forests (RF) were used for model construction.
Results:
There were 258 patients (female, n=96, male, n=162; low-risk, n=28, high-risk, n=230), with a mean age of 60±17 years. Adverse outcomes: low-risk, n=4(14.3%)l high-risk, n=34 (14.7%).
The non-validated BLR model demonstrated three significant predictive factors: neurological disorder (p=0.03), stent on strings insertion (p=0.04) and complete stone clearance (p=0.04). Model accuracy=0.90 (95% CI:0.85-0.93), sensitivity=0.90, specificity=0.80. There were no significant differences between the low/high risk groups (p=0.14).
The internally validated RF model demonstrated two significant predictive factors (history of post-operative UTI, p=0.004; neurological disorder, p<0.001). Model accuracy=0.88 (95% CI: 0.77-0.94), sensitivity=1.00, specificity=0.00, AUC=0.71. Figure for literature comparison.
Conclusions:
Presence of a neurological disorder is consistent risk factor for post-ureteroscopy infective complications. Modern machine learning techniques fail to accurately predict those with an infective complication.
Introduction:
Post ureteroscopic infectious complications have proven difficult to predict. We therefore aimed to use described risk factors to delineate low and high risk groups to compare risk of post ureteroscopic infective complications.
Materials and Methods:
Retrospective cohort of all patients undergoing ureteroscopy from June 2019 to September 2019. Data collected on age, sex, type of admission, presence of a stent, operative factors and the presence of risk factors (diabetes mellitus, neurological disorders, immunocompromised state, abnormal upper tract anatomy, previous reconstructive surgery, previous post-operative UTI). Patients were divided into low(no risk factors)/high risk groups. Adverse outcome defined as post-operative pyrexia, ITU/HDU admission for urosepsis and/or 30 day readmission for infective symptoms. Binary logistic regression (BLR) and random forests (RF) were used for model construction.
Results:
There were 258 patients (female, n=96, male, n=162; low-risk, n=28, high-risk, n=230), with a mean age of 60±17 years. Adverse outcomes: low-risk, n=4(14.3%)l high-risk, n=34 (14.7%).
The non-validated BLR model demonstrated three significant predictive factors: neurological disorder (p=0.03), stent on strings insertion (p=0.04) and complete stone clearance (p=0.04). Model accuracy=0.90 (95% CI:0.85-0.93), sensitivity=0.90, specificity=0.80. There were no significant differences between the low/high risk groups (p=0.14).
The internally validated RF model demonstrated two significant predictive factors (history of post-operative UTI, p=0.004; neurological disorder, p<0.001). Model accuracy=0.88 (95% CI: 0.77-0.94), sensitivity=1.00, specificity=0.00, AUC=0.71. Figure for literature comparison.
Conclusions:
Presence of a neurological disorder is consistent risk factor for post-ureteroscopy infective complications. Modern machine learning techniques fail to accurately predict those with an infective complication.
Post ureteroscopic infectious complications have proven difficult to predict. We therefore aimed to use described risk factors to delineate low and high risk groups to compare risk of post ureteroscopic infective complications.
Materials and Methods:
Retrospective cohort of all patients undergoing ureteroscopy from June 2019 to September 2019. Data collected on age, sex, type of admission, presence of a stent, operative factors and the presence of risk factors (diabetes mellitus, neurological disorders, immunocompromised state, abnormal upper tract anatomy, previous reconstructive surgery, previous post-operative UTI). Patients were divided into low(no risk factors)/high risk groups. Adverse outcome defined as post-operative pyrexia, ITU/HDU admission for urosepsis and/or 30 day readmission for infective symptoms. Binary logistic regression (BLR) and random forests (RF) were used for model construction.
Results:
There were 258 patients (female, n=96, male, n=162; low-risk, n=28, high-risk, n=230), with a mean age of 60±17 years. Adverse outcomes: low-risk, n=4(14.3%)l high-risk, n=34 (14.7%).
The non-validated BLR model demonstrated three significant predictive factors: neurological disorder (p=0.03), stent on strings insertion (p=0.04) and complete stone clearance (p=0.04). Model accuracy=0.90 (95% CI:0.85-0.93), sensitivity=0.90, specificity=0.80. There were no significant differences between the low/high risk groups (p=0.14).
The internally validated RF model demonstrated two significant predictive factors (history of post-operative UTI, p=0.004; neurological disorder, p<0.001). Model accuracy=0.88 (95% CI: 0.77-0.94), sensitivity=1.00, specificity=0.00, AUC=0.71. Figure for literature comparison.
Conclusions:
Presence of a neurological disorder is consistent risk factor for post-ureteroscopy infective complications. Modern machine learning techniques fail to accurately predict those with an infective complication.
Code of conduct/disclaimer available in General Terms & Conditions
{{ help_message }}
{{filter}}