Routine urinary biochemistry does not accurately predict stone type nor recurrence in kidney stone
formers: A multi-centre, multi-model, externally validated machine-learning study
BAUS ePoster online library. Geraghty R. 06/21/21; 319102; p6-4
Disclosure(s): I am an academic clinical fellow supported by NIHR
formers: A multi-centre, multi-model, externally validated machine-learning study
Mr. Robert Geraghty
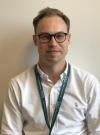
REGULAR CONTENT
Login now to access Regular content available to all registered users.
Abstract
Discussion Forum (0)
Rate & Comment (0)
Introduction:
Urinary biochemistry is used to detect and monitor conditions associated with recurrent kidney stones. There are no predictive machine learning (ML) tools for kidney stone type or recurrence. We therefore aimed to build and validate ML models for these outcomes using age, gender, 24-hour urine biochemistry and stone composition.
Materials and Methods:
Patient data from 3 large tertiary referral centres were used (n=9791), from the UK and Switzerland. Of these 3130 had available 24-hour urine biochemistry measurements (calcium, oxalate, urate, pH, volume), and 1684 had clinical data on kidney stone recurrence. Predictive models were constructed using two ML techniques (Partitioning and Random Forests [RF]) and validated with internal/external datasets.
Results:
See figure for demographics of included patients. For kidney stone type, on external validation accuracy of UK RF model=0.79 (95% CI: 0.73-0.84), sensitivity: calcium oxalate=0.99 and calcium phosphate/urate=0.00. Specificity: calcium oxalate=0.00 and calcium phosphate/urate=0.99. For the Swiss RF model accuracy=0.87 (95% CI:0.83-0.89), sensitivity: calcium oxalate=0.99 and calcium phosphate/urate=0.00. Specificity: calcium oxalate=0.00, calcium phosphate=0.00 and urate=1.00.
For stone recurrence, on external validation accuracy of UK RF model=0.22 (95% CI: 0.19-0.25),
sensitivity=0.93 and specificity=0.09. Swiss RF model accuracy=0.42 (95% CI: 0.39-0.47), sensitivity=0.03 and specificity=0.97.
Conclusion:
Neither kidney stone type nor kidney stone recurrence can be accurately predicted using modelling tools built using specific 24-hour urinary biochemistry values alone. Further studies to delineate accurate predictive tools should be undertaken using both known and novel risk factors.
Urinary biochemistry is used to detect and monitor conditions associated with recurrent kidney stones. There are no predictive machine learning (ML) tools for kidney stone type or recurrence. We therefore aimed to build and validate ML models for these outcomes using age, gender, 24-hour urine biochemistry and stone composition.
Materials and Methods:
Patient data from 3 large tertiary referral centres were used (n=9791), from the UK and Switzerland. Of these 3130 had available 24-hour urine biochemistry measurements (calcium, oxalate, urate, pH, volume), and 1684 had clinical data on kidney stone recurrence. Predictive models were constructed using two ML techniques (Partitioning and Random Forests [RF]) and validated with internal/external datasets.
Results:
See figure for demographics of included patients. For kidney stone type, on external validation accuracy of UK RF model=0.79 (95% CI: 0.73-0.84), sensitivity: calcium oxalate=0.99 and calcium phosphate/urate=0.00. Specificity: calcium oxalate=0.00 and calcium phosphate/urate=0.99. For the Swiss RF model accuracy=0.87 (95% CI:0.83-0.89), sensitivity: calcium oxalate=0.99 and calcium phosphate/urate=0.00. Specificity: calcium oxalate=0.00, calcium phosphate=0.00 and urate=1.00.
For stone recurrence, on external validation accuracy of UK RF model=0.22 (95% CI: 0.19-0.25),
sensitivity=0.93 and specificity=0.09. Swiss RF model accuracy=0.42 (95% CI: 0.39-0.47), sensitivity=0.03 and specificity=0.97.
Conclusion:
Neither kidney stone type nor kidney stone recurrence can be accurately predicted using modelling tools built using specific 24-hour urinary biochemistry values alone. Further studies to delineate accurate predictive tools should be undertaken using both known and novel risk factors.
Introduction:
Urinary biochemistry is used to detect and monitor conditions associated with recurrent kidney stones. There are no predictive machine learning (ML) tools for kidney stone type or recurrence. We therefore aimed to build and validate ML models for these outcomes using age, gender, 24-hour urine biochemistry and stone composition.
Materials and Methods:
Patient data from 3 large tertiary referral centres were used (n=9791), from the UK and Switzerland. Of these 3130 had available 24-hour urine biochemistry measurements (calcium, oxalate, urate, pH, volume), and 1684 had clinical data on kidney stone recurrence. Predictive models were constructed using two ML techniques (Partitioning and Random Forests [RF]) and validated with internal/external datasets.
Results:
See figure for demographics of included patients. For kidney stone type, on external validation accuracy of UK RF model=0.79 (95% CI: 0.73-0.84), sensitivity: calcium oxalate=0.99 and calcium phosphate/urate=0.00. Specificity: calcium oxalate=0.00 and calcium phosphate/urate=0.99. For the Swiss RF model accuracy=0.87 (95% CI:0.83-0.89), sensitivity: calcium oxalate=0.99 and calcium phosphate/urate=0.00. Specificity: calcium oxalate=0.00, calcium phosphate=0.00 and urate=1.00.
For stone recurrence, on external validation accuracy of UK RF model=0.22 (95% CI: 0.19-0.25),
sensitivity=0.93 and specificity=0.09. Swiss RF model accuracy=0.42 (95% CI: 0.39-0.47), sensitivity=0.03 and specificity=0.97.
Conclusion:
Neither kidney stone type nor kidney stone recurrence can be accurately predicted using modelling tools built using specific 24-hour urinary biochemistry values alone. Further studies to delineate accurate predictive tools should be undertaken using both known and novel risk factors.
Urinary biochemistry is used to detect and monitor conditions associated with recurrent kidney stones. There are no predictive machine learning (ML) tools for kidney stone type or recurrence. We therefore aimed to build and validate ML models for these outcomes using age, gender, 24-hour urine biochemistry and stone composition.
Materials and Methods:
Patient data from 3 large tertiary referral centres were used (n=9791), from the UK and Switzerland. Of these 3130 had available 24-hour urine biochemistry measurements (calcium, oxalate, urate, pH, volume), and 1684 had clinical data on kidney stone recurrence. Predictive models were constructed using two ML techniques (Partitioning and Random Forests [RF]) and validated with internal/external datasets.
Results:
See figure for demographics of included patients. For kidney stone type, on external validation accuracy of UK RF model=0.79 (95% CI: 0.73-0.84), sensitivity: calcium oxalate=0.99 and calcium phosphate/urate=0.00. Specificity: calcium oxalate=0.00 and calcium phosphate/urate=0.99. For the Swiss RF model accuracy=0.87 (95% CI:0.83-0.89), sensitivity: calcium oxalate=0.99 and calcium phosphate/urate=0.00. Specificity: calcium oxalate=0.00, calcium phosphate=0.00 and urate=1.00.
For stone recurrence, on external validation accuracy of UK RF model=0.22 (95% CI: 0.19-0.25),
sensitivity=0.93 and specificity=0.09. Swiss RF model accuracy=0.42 (95% CI: 0.39-0.47), sensitivity=0.03 and specificity=0.97.
Conclusion:
Neither kidney stone type nor kidney stone recurrence can be accurately predicted using modelling tools built using specific 24-hour urinary biochemistry values alone. Further studies to delineate accurate predictive tools should be undertaken using both known and novel risk factors.
Code of conduct/disclaimer available in General Terms & Conditions
{{ help_message }}
{{filter}}